Revolutionizing Electronic Design and Manufacturing: The Power of AI Technology
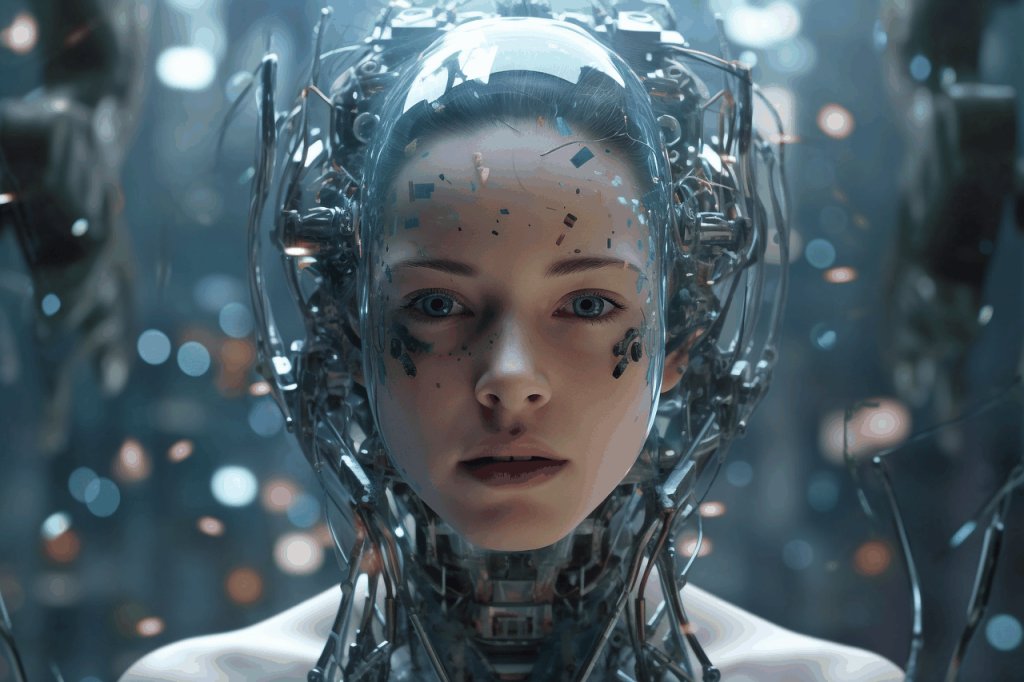
In recent years, the integration of Artificial Intelligence (AI) technology into various industries has revolutionized processes and improved efficiency. The electronic design and manufacturing sector is no exception. With its ability to analyze vast amounts of data, learn patterns, and make intelligent decisions, AI has become a game-changer in optimizing electronic design, enhancing manufacturing processes, and driving innovation. In this article, we will explore in detail the significant impact of AI technology on electronic design and manufacturing.
Design Optimization:
AI technology has transformed the electronic design process by enabling advanced optimization techniques. Designers can leverage AI algorithms to explore a vast design space, quickly evaluate numerous possibilities, and identify the most efficient and effective designs. AI algorithms can analyze design constraints, performance objectives, and historical data to generate optimized circuit layouts, improve power efficiency, reduce electromagnetic interference, and enhance overall design performance.
For example, AI-based optimization algorithms can analyze a wide range of circuit parameters and constraints to find the optimal trade-off between power consumption, performance, and cost. These algorithms can identify design solutions that human designers might overlook, leading to more efficient and innovative electronic designs.
Predictive Maintenance:
In the manufacturing phase, AI technology plays a crucial role in predictive maintenance. By continuously monitoring sensors and data points, AI algorithms can detect anomalies, predict equipment failures, and recommend preventive actions. This proactive approach minimizes unplanned downtime, reduces maintenance costs, and optimizes production efficiency.
AI-powered predictive maintenance algorithms can analyze real-time data, historical records, and equipment performance patterns to accurately forecast maintenance requirements and schedule interventions accordingly. For example, by monitoring equipment vibration, temperature, and power consumption data, AI algorithms can identify signs of impending equipment failure and alert maintenance teams to take preventive action. This ensures that equipment operates at peak performance, reduces costly downtime, and extends the lifespan of machinery.
Quality Control and Inspection:
AI technology enhances quality control and inspection processes in electronic manufacturing. Visual inspection, a critical aspect of quality control, benefits from AI-driven machine vision systems. These systems employ deep learning algorithms to analyze images and detect defects, such as soldering errors, component misalignment, or physical damage. By automating the inspection process, AI technology improves accuracy, reduces human error, and increases throughput, ultimately ensuring higher product quality.
AI-powered machine vision systems can quickly and accurately identify defects that may be difficult for human inspectors to detect. This technology not only improves the efficiency of quality control but also reduces the likelihood of defective products reaching the market, thereby enhancing customer satisfaction and brand reputation.
Supply Chain Optimization:
AI technology offers immense value in optimizing the electronic manufacturing supply chain. By analyzing historical data, market trends, and demand patterns, AI algorithms can accurately forecast material requirements, manage inventory levels, and optimize procurement processes. This helps to minimize supply chain disruptions, reduce excess inventory, lower costs, and enhance overall efficiency.
AI algorithms can analyze vast amounts of data to identify patterns and correlations, enabling more accurate demand forecasting. By leveraging this information, manufacturers can optimize their production schedules, avoid stockouts, and reduce inventory carrying costs. Additionally, AI-powered algorithms can continuously monitor market trends and supplier performance, allowing manufacturers to make informed decisions regarding sourcing strategies and supplier selection.
Process Automation:
AI technology enables process automation in electronic manufacturing, improving productivity and reducing manual labor. Robotic process automation (RPA) powered by AI algorithms can streamline repetitive and time-consuming tasks, such as data entry, documentation, and inventory management. This automation allows human workers to focus on higher-value tasks, leading to increased productivity, reduced errors, and improved overall workflow efficiency.
RPA can automate various tasks across the manufacturing process, such as generating production reports, updating inventory databases, or performing quality control checks.
Revolutionizing Electronic Design and Manufacturing: The Power of AI Technology Read More »